The intersection of digital innovation and agriculture presents numerous opportunities for enhancing farming efficiency and sustainability. One of the most compelling technological advancements in this area is the application of digital twins. Digital twins in agriculture refer to virtual models of farming systems, processes, or products. These models, continuously updated with real-time data, enable farmers to make precise decisions, optimize processes, and predict future outcomes.
1. Introduction to Digital Twins
2. What are Digital Twins?
3. How Digital Twins are Transforming Agriculture & Usecases
4. Case Studies and Current Applications of Digital Twins
5. Step-by-Step Implementation of Digital Twins in Farming
6. Challenges and Considerations
7. The Future of Digital Twins in Agriculture
By integrating digital twins into agricultural practices, farmers can visualize complex data, simulate the impact of different choices on crop yields, and ultimately manage resources more effectively. This approach not only improves productivity but also contributes significantly to the sustainability of agricultural operations, addressing both environmental impacts and operational efficiency.
As agriculture faces the challenges of climate change, resource scarcity, and increased food demand, digital twins serve as a pivotal tool in adapting and thriving under these pressures. The use of this technology fosters a deeper understanding of the intricate interactions within farming environments, leading to more informed decisions and resilient agricultural systems.
With digital twins, agricultural professionals have the capability to oversee and adjust their operations like never before. This emerging technology is reshaping how knowledge is applied in farming, transitioning from traditional practices to a more data-driven, precise agriculture.
Purcell and Neubauer (2022) note,
“Digital Twins represent a paradigm shift in how we can dynamically understand and interact with agriculture, providing a virtual representation that is updated from real-time data”.
Further emphasizing the significance of this innovation, the Fraunhofer IESE (2020) highlights,
“The introduction of digital twins in agriculture marks a significant advancement towards Agriculture 4.0, integrating cyber-physical systems into farming practices”.
These insights underline the transformative impact digital twins have on the agricultural sector.
2. What are Digital Twins?
A digital twin is a virtual model designed to accurately reflect a physical object, system, or process. This technology integrates real-time data, simulation, machine learning, and reasoning to enhance decision-making processes across various industries. By mirroring a physical object or system, digital twins allow businesses to simulate, predict, and optimize the lifecycle of products or processes without physically interacting with them.
Core Components of Digital Twins
Sensors and IoT Devices: These components collect real-time data from their physical counterparts. They play a crucial role in ensuring that the digital twin’s data is reflective of the current state of the physical object or system.
Data Analytics Platforms: These platforms process and analyze the data collected by sensors to generate insights. They enable users to understand and predict the behavior of the physical counterpart, facilitating informed decision-making.
Simulation Models: Simulation models use the insights generated by data analytics to replicate how the physical object or system will behave under various conditions. This is crucial for testing, optimization, and forecasting.
Integration with Advanced Technologies
Digital twins are significantly enhanced through integration with advanced technologies:
Internet of Things (IoT) enables seamless communication between the digital twin and its physical counterpart.
Artificial Intelligence (AI) and Machine Learning (ML) provide predictive analytics and adaptive learning capabilities to simulate different scenarios and adapt to changing conditions.
Cloud Computing offers the scalability needed to handle large sets of data and complex simulations.
Industry Applications and Examples. Digital twins have been applied across various sectors:
- Healthcare: Companies like Pfizer use digital twins for drug development and testing, allowing them to simulate the behavior of drugs across various scenarios without physical trials (DigiTwins Consulting) (XB Software).
- Manufacturing: In industries such as automotive and aerospace, digital twins optimize production processes and design, improving efficiency and reducing the need for physical prototypes.
- Urban Planning and Infrastructure: They are used to model cities and infrastructure projects, helping planners optimize layouts and predict the impacts of different scenarios (Thales Group).
Benefits of Digital Twins
The implementation of digital twins offers numerous benefits:
- Enhanced Decision-Making: By providing a comprehensive view of how systems and processes operate under various conditions, digital twins allow companies to make more informed decisions (Matterport).
- Increased Efficiency: They help in optimizing processes, reducing downtime through predictive maintenance, and saving costs by eliminating the need for physical prototypes (McKinsey & Company).
- Improved Product Quality and Innovation: Digital twins facilitate the continuous improvement and innovation of products by allowing the simulation of new ideas and identification of potential problems before they occur.
By creating a dynamic digital representation of physical assets, digital twins enable a deeper understanding of systems, enhance operational efficiency, and improve the predictability of outcomes as stated by sources like Agrimetrics and researchers Purcell and Neubauer (Matterport).
3. How Digital Twins are Transforming Agriculture & Usecases
Digital twins in agriculture represent a critical evolution in the intersection of information technology and traditional farming practices. By creating dynamic, real-time virtual models of physical farm assets—whether these be individual plants, livestock, fields, or entire ecosystems—digital twins facilitate a deep understanding and precise management of agricultural operations. They leverage data from a myriad of sources including IoT sensors, satellites, drones, and weather stations, and apply advanced algorithms to optimize the decision-making process in farming.
Use Cases of Digital Twins in Agriculture
- Precision Agriculture and Yield Optimization
In the realm of precision agriculture, digital twins excel by integrating and analyzing data at an unprecedented scale. For instance, digital models of crop fields can simulate the impact of various farming interventions on crop yield under different weather scenarios. This predictive capability allows farmers to make more informed decisions about when to plant, irrigate, fertilize, and harvest. As highlighted by Agrimetrics, the application of digital twins has led to
“precise monitoring and management of crop health, resulting in a 20% increase in yield,”
showcasing how data-driven strategies can directly enhance productivity and profitability.
Read more about precision agriculture.
- Resource Management and Sustainability
Digital twins also address critical sustainability challenges by ensuring optimal resource utilization. They can manage the distribution and use of water and chemicals more efficiently, minimizing runoff and the environmental impact of farming. By closely monitoring soil conditions and crop health, these models can dictate the precise amount of water and nutrients needed, considerably reducing waste and enhancing the sustainability of farming practices.
- Livestock Management
In livestock management, digital twins help in monitoring health and productivity by collecting data through sensors attached to the animals. These twins can track movement, behavior, and vital signs to provide early warning of health issues, improving animal welfare and reducing mortality rates. This technology enables farmers to tailor animal husbandry practices to the needs of individual animals, thereby optimizing their health and productivity.
- Farm Equipment and Machinery Optimization
The use of digital twins extends to farm machinery and equipment, where they facilitate predictive maintenance and operational efficiency. By analyzing data from tractors and other machinery, digital twins predict when a piece of equipment is likely to fail and suggest preventive maintenance. This not only helps in avoiding costly downtime but also extends the life of the equipment.
- Supply Chain and Market Access
On a broader scale, digital twins assist in streamlining the agricultural supply chain. They can simulate market demand and supply fluctuations to help farmers plan their crop production more effectively. This aligns production more closely with market demand, reducing surplus and shortages, and improving profitability.
- Environmental Impact and Regulatory Compliance
Digital twins also play a vital role in environmental monitoring and regulatory compliance. They can track and verify farming practices against environmental regulations and standards, ensuring that farming practices are sustainable and compliant with local and international laws.
- Integration with Broader Digital Ecosystems
Finally, digital twins do not operate in isolation. They are often integrated into broader digital ecosystems, incorporating data from adjacent sectors such as meteorology, hydrology, and market economics. This integration enables a holistic approach to agricultural management that not only focuses on maximizing yield and profit but also on sustaining resources for future generations.
As the Digital Twin Consortium suggests,
“Digital twins serve as a cornerstone for innovation in agricultural processes, providing a platform for experimentation without risk.”
This encapsulates the broader value proposition of digital twins in agriculture, highlighting their role as pivotal tools for risk mitigation, innovation, and continuous improvement in the agricultural sector.
4. Case Studies and Current Applications of Digital Twins in Agriculture
Digital Twin Consortium
The Digital Twin Consortium is pioneering the integration of digital twin technology across various sectors, including agriculture. Their focus is on utilizing digital twins to address challenges in the food and beverage sectors, enhancing sustainability and supply chain efficiency. By simulating and analyzing the lifecycle of agricultural products from production to consumption, the consortium’s initiatives help optimize supply chains, reduce waste, and improve food safety. These digital twins are critical for monitoring crop conditions and predicting outcomes, facilitating innovation and risk-free experimentation in agricultural processes.
Agrimetrics
Agrimetrics has harnessed digital twins to significantly enhance farming practices. They have implemented digital twins in the UK for optimizing wheat production by integrating data from soil sensors, weather reports, and satellite imagery. This comprehensive digital twin model enables real-time monitoring and scenario simulation, allowing for precise irrigation and fertilization adjustments. The result is a significant increase in yield, with Agrimetrics reporting a “20% increase in yield,” underscoring the effectiveness of digital twins in boosting crop health and optimizing resource allocation. About Agrimetrics.
Intellias
Intellias applies digital twin technology to streamline various farming operations, including field monitoring and climate risk management. They focus on creating efficient, controlled farming environments through precision agriculture techniques. By using digital twins, Intellias aids farmers in optimizing resource use—such as water and fertilizers—thereby reducing waste and enhancing sustainable practices. Their digital twins also support weather impact predictions and climate risk management, ensuring better crop resilience and yield predictability. Read more about Intellias and how to implement digital twins.
SEKAI
While primarily known for its work with wind turbines, SEKAI offers valuable insights into how digital twin technology could be adapted for agricultural use. Their methodology, which includes real-time data gathering and predictive maintenance, could be beneficial in agricultural settings where equipment efficiency and environmental conditions are crucial for crop success. This adaptation illustrates the versatility of digital twins and their potential beyond their initial applications.
These case studies demonstrate the transformative potential of digital twins in agriculture. Through detailed analysis and simulation, digital twins equip agricultural professionals with advanced tools to enhance productivity, sustainability, and resource efficiency, marking a substantial advancement towards more innovative and resilient farming systems.
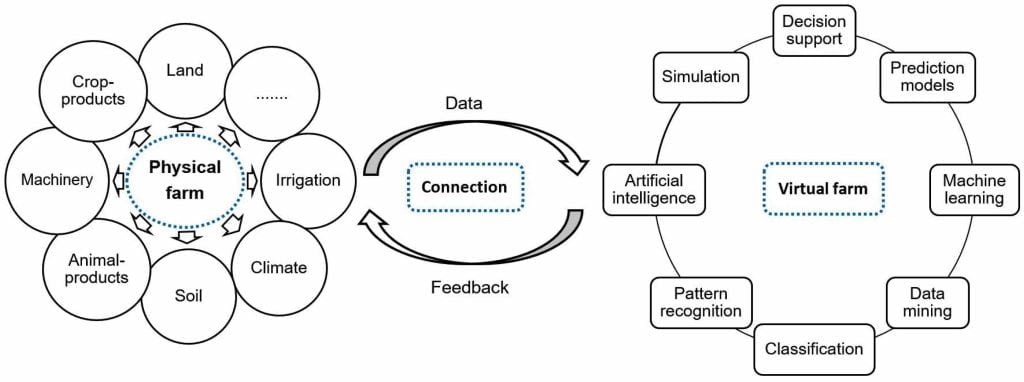
Concept of a Digital Twin in Agriculture
5. Step-by-Step Implementation of Digital Twins in Farming
Implementing digital twins in agriculture is a transformative process that leverages advanced technology to mirror and enhance the physical operations of farming. This step-by-step guide provides detailed guidance on how to effectively deploy digital twins from conceptualization to execution.
Step 1: Infrastructure Setup
Setting up the infrastructure for digital twins in agriculture is the critical first step, involving the deployment of necessary hardware and connectivity solutions that will form the backbone of your digital twin system.
Key Hardware Requirements:
- Sensors: Deploy a variety of sensors across the farm to continuously monitor vital parameters such as soil moisture levels, temperature, pH levels, and crop health indicators. These sensors form the primary data collection points that feed into the digital twin system.
- IoT Devices: Implement robust IoT devices that facilitate the seamless transmission of sensor data. These devices should be capable of operating in diverse agricultural environments and resilient against weather conditions and other physical disruptions.
- Connectivity Infrastructure: Establish a reliable connectivity framework using technologies such as Wi-Fi, LoRaWAN, or cellular networks. This infrastructure must ensure that data collected from various points on the farm is transmitted in real time or at scheduled intervals without loss.
Step 2: Data Collection and Management
Effective data collection and management are paramount for the success of digital twins in farming. This step ensures that the data flowing from the field is accurate, timely, and securely handled.
Data Management Strategies:
- Data Acquisition Protocols: Define clear protocols for how data is to be collected, including the frequency of data capture and the specific data points that are required for effective modeling.
- Data Integration: Integrate data from various sources, including weather stations, farm equipment, and external data services to enrich the digital twin’s dataset.
- Data Security and Integrity: Implement robust security measures to protect data from unauthorized access and ensure its integrity. This may include encryption of data transmissions and secure storage solutions.
Step 3: Model Development and Integration
Developing an accurate model of the physical farm is essential for the digital twin to function as an effective decision-making tool. This model should integrate seamlessly with real-time data and update dynamically to reflect current conditions.
Modeling Techniques:
- Simulation Models: Develop detailed simulation models that can predict future farm conditions based on current data inputs. This could include growth models for crops under various weather conditions and pest invasion scenarios.
- Machine Learning Models: Utilize machine learning algorithms to enhance the predictive accuracy of your digital twin. These models can learn from historical data to improve their forecasts and operational suggestions.
Step 4: Analysis and Application
The final step involves analyzing the collected data and model outputs to make informed decisions that enhance farm operations and productivity.
Analytical Applications:
- Predictive Analytics: Use the digital twin to perform predictive analytics that forecast crop yields, pest attacks, and resource needs, allowing for proactive farm management.
- Real-Time Decision Making: Leverage the real-time data and dynamic modeling capabilities of the digital twin to make immediate operational decisions, such as irrigation adjustments and resource allocation, based on current farm conditions.
Benefits:
- Increased Operational Efficiency: Through continuous monitoring and predictive analytics, digital twins help streamline farm operations, reduce waste, and optimize resource use.
- Enhanced Crop Yields: By predicting and mitigating risks before they impact crop health, digital twins can significantly improve crop yields and overall farm productivity.
Implementing digital twins in agriculture is a complex but rewarding endeavor that can significantly enhance the efficiency and output of farming operations. By following these detailed steps, farmers and agricultural managers can ensure they fully harness the potential of this cutting-edge technology.
6. Challenges and Considerations
Implementing digital twins in agriculture involves navigating a complex landscape of technical, security, and ethical issues. Each aspect must be meticulously managed to ensure the effectiveness and integrity of digital twin technologies in enhancing farming operations.
Technical Challenges
The success of digital twins hinges on the precision and reliability of the data they utilize. As noted by Purcell and Neubauer in 2022,
“The accuracy of digital twins depends on the quality of the data collected, posing a significant challenge in ensuring reliable models.”
To address this, rigorous calibration of sensors, consistent data validation practices, and the integration of diverse data sources are essential to mitigate any single point of data failure.
System integration also presents a significant hurdle. Ensuring that various technological components such as sensors, IoT devices, data management platforms, and user interfaces work seamlessly together requires a modular system design that allows for easier maintenance and scalability.
Cybersecurity Measures
With the centralization of sensitive farm data, implementing robust cybersecurity measures is crucial to protect against unauthorized access and cyber threats. This includes end-to-end encryption for data transmissions, regular security audits, and adherence to international data protection regulations. Additionally, training staff on cybersecurity best practices is vital for preventing data breaches and enhancing system resilience.
Ethical and Privacy Concerns
The utilization of digital twins raises important questions about the ownership and use of agricultural data. As highlighted by experts from Fraunhofer IESE in 2020,
“While digital twins offer substantial benefits, they require careful consideration of data privacy and security to ensure that farmers’ rights are protected.”
Ensuring that farmers retain ownership and control over their data involves transparent data handling practices and the implementation of consent protocols before data collection.
Moreover, protecting the privacy of farm data is not only about securing it against threats but also ensuring that it is not exploited unfairly. Implementing data anonymization techniques and strict access controls are essential steps in protecting individual privacy and preventing misuse.
Navigating these challenges effectively requires a balanced approach that prioritizes technical efficacy, robust security measures, and ethical considerations. By addressing these issues diligently, the agricultural sector can fully leverage the transformative benefits of digital twins while safeguarding the interests and data integrity of farmers. This careful consideration will help foster trust and encourage the wider adoption of digital twin technologies across the agricultural industry.
7. The Future of Digital Twins in Agriculture
The evolution of digital twin technologies in agriculture is increasingly being recognized as a transformative force capable of reshaping the agricultural landscape. As these technologies integrate more deeply with emerging technologies such as AI and automated systems, their potential to enhance decision-making, operational efficiency, and sustainability is significantly amplified.
Technological Advancements and Integration
Digital twins, by creating a digital replica of farming operations, allow for the seamless decoupling of physical processes from their management and control. This capability facilitates remote management of agricultural operations based on real-time digital information rather than direct manual intervention, leading to more precise and timely decision-making. Verdouw and his colleagues emphasize the transformative nature of this integration:
“Using Digital Twins as a central means for farm management enables the decoupling of physical flows from its planning and control”.
As AI technologies become more integrated, digital twins will play a crucial role in agriculture. Purcell and Neubauer highlight this trend, stating, “As AI integration deepens, digital twins will become even more pivotal in driving the next wave of agricultural innovation”.
Scalability and Global Impact
The scalability of digital twins means their benefits can be extended from small farms to large agricultural enterprises, enhancing global agricultural practices. This scalability not only promises increased operational efficiency and reduced environmental impact but also supports the broader adoption of sustainable practices across different agricultural contexts. Researchers from Fraunhofer IESE suggest that
“Future advancements in digital twins will likely focus on enhancing their predictive capabilities and expanding their applicability across more aspects of agriculture”,
indicating the vast potential of digital twins to revolutionize the field.
Challenges and Continuous Advancement
While the prospects are promising, the journey towards fully realizing the potential of digital twins in agriculture is not devoid of challenges. The accuracy of these systems heavily depends on the data quality and integration capabilities, which are essential for creating reliable models that accurately mirror real-world conditions. Continuous advancements in IoT and AI technologies are critical in addressing these challenges, enhancing the predictive capabilities of digital twins, and expanding their applicability across all aspects of agriculture.
Encouraging Broad Adoption
The agricultural sector is encouraged to embrace the adoption and integration of digital twin technologies actively. Doing so will unlock new dimensions of efficiency and sustainability, propelling the sector towards more advanced, precision-based farming practices. The transformative potential of digital twins extends beyond mere operational enhancements; it promises a revolutionary shift towards more sustainable and productive agricultural systems globally.
The adoption of digital twin technology in agriculture represents not just a step forward in technological integration but a significant leap towards a future where farming is smarter, more connected, and sustainably aligned with global food system demands. As Purcell and Neubauer aptly put it,
“Embracing digital twins can lead to revolutionary changes in agriculture, making it more efficient, sustainable, and profitable”.
As this technology continues to evolve and adapt, it will undoubtedly play a pivotal role in shaping the next generation of agricultural practices, making them more responsive to the rapidly changing global landscapes.